Snapshot
One of California’s largest utilities developed machine learning models on-prem to enable asset-level wildfire risk prediction during emergency activations. Logic20/20 partnered with this utility to (1) design a production-grade AWS serverless cloud architecture and (2) migrate models into the cloud production environment. These efforts greatly enhanced the efficiency, reliability, transparency, and sustainability of the model application and enabled the business to integrate machine learning insights into grid planning, emergency response, public safety, and asset protection efforts.
Our team built production-grade predictive model applications that are scheduled to run in the cloud daily—or more often if emergency conditions arise. Today our client has data-driven ignition and failure risk predictions ready for use in emergency decision-making at a moment’s notice.
We brought our expertise and experience in:
-
Agile methodologies
-
Machine Learning Operations (MLOps)
-
Data science excellence
-
Cloud transformation
-
Software and data engineering
-
AWS Services
A Golden State power player
Our client is one of California’s largest gas and electric utility providers, delivering energy to more than 3.5 million customers across a service area of over 4,000 miles. They have served their area for more than a century and currently employ over 4,500 Californians.
Putting data to work for emergency decision making
The utility’s internal data scientists developed a system of machine learning models that predict asset ignition and failure risk to inform public safety power shutoff (PSPS) decisions. These sophisticated models incorporate real-time data such as temperature and wind speed at the pole-and-span level to predict if and when an asset could ignite or fail. In an emergency situation, the resulting insights prepare the utility to determine whether power should be shut off for safety purposes, and if so, when, where, and for how long.
A major pain point of the solution on-prem was the time and manual effort required to produce reliable results from the model. These challenges made it difficult for the model to be used during emergency activations and compromised the organization’s investment in machine learning and progress against its goal to make the customers in its service area safer. To run more quickly and reliably on cloud, a flexible inference pipeline was needed as well as an architecture that could efficiently orchestrate the complex data inputs, outputs, and logical processes that underpin the machine learning system.
Building a high-performance production environment
Logic20/20 used Agile principles to structure a development team of five machine learning engineers and data scientists, a scrum master, data science architect, and a product owner from the client side who closely integrated to form a hybrid team. First, they executed the migration of the models from on-premise servers to an AWS serverless architecture. Within two quarters they took the utility from having no AWS footprint to having a three-environment production architecture and completed their first in-production run.
Once the migration was complete, our team turned their efforts towards optimization and completed a major re-architecture of the inference pipeline.
Today our client’s serverless AWS architecture includes
• Independent feature ETL for transparency and feature sharing
• Automated audit trail
• Model and data versioning
• Cost tracking and transparency
• Scheduling and failure notifications
• Automated result sharing with stakeholders and applications
• Grid investment model consumable via API
• Flexible, reusable inference pipeline that can support different model types
Building a safer future for California communities
Today our client’s ignition prediction models run in a secure, auditable production environment that delivers on the benefits of cloud computing: reduced costs, greater flexibility and scalability, enhanced shareability with other teams, and access to a broad range of cloud-based services. The new environment also reduces the amount of time required to bring new and updated models into production and delivers increased auditability and transparency.
The data science workbench and productionized models our team built are now supporting not only PSPS decisions, but also grid investment planning. Our client can use outputs from these models to identify areas where assets are at highest risk for failure—and where those failures would have the greatest impact—to optimize use of their investment dollars in ensuring a safe, reliable grid. The result is increased safety for communities over the short and long term as well as the chance to allocate investment dollars where they can deliver the greatest benefit.
Want to start a project like this?
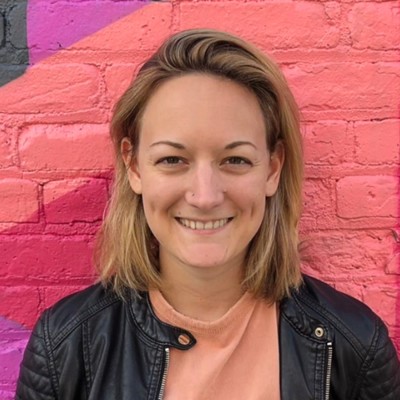
Kaitlyn Petronglo is a Manager in the Advanced Analytics practice area at Logic20/20.